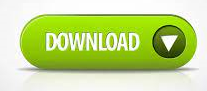
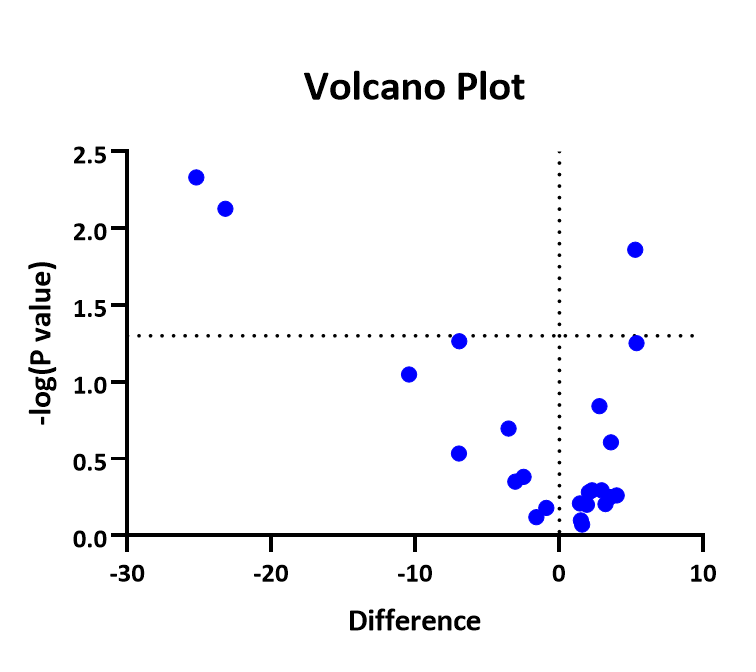
No other software offers curve fitting and other statistical analysis to be so easy, comprehensive, correct and simple! Wide Range of Applications It facilitates the choice of the statistical tests and help you to interpret the results. GraphPad Prism guides you through the process of the analysis. Many undergraduate and graduate students also use GraphPad Prism. Meanwhile Graphpad Prism is used throughout the life sciences sector. After all, do I 'really' just want to care about one gene? What if a large number of genes are differentially expressed (most moreso than my gene of interest)? Is my gene of interest really 'special' then? I guess it depends on what your question is but it's something to think about.GraphPad Prism was originally developed for experimental biologists, medicine scientists and pharmacologists.

What will a p-value (calculated based on assumptions that really aren't exactly true) in this case really tell you? That said, if you have no other options and if you really believe that your gene of interest is differentially expressed and that despite all the limitations of microarray, it has enough power to detect the effect if it exists, then go for it.įor me, I usually end up caring about more genes than just a single gene of interest when I have genome-wide data available (so I practically almost always use FDR control). Not sure how much info you can gain from it.

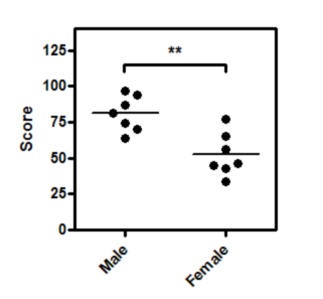
You're trying to validate a gene of interest using an assay that is fairly crappy for differential gene expression of individual genes. There are a lot of concepts involved here (noise, dynamic range, etc.) but essentially it boils down to: microarrays tell you which genes (out of tens of thousands) are likely to be differentially expressed whereas qPCR is much better at telling you whether a single of gene interest is likely to be differentially expressed. However, keep in mind that using microarray to analyze a single gene isn't ideal because that's not what microarrays were designed for (they were designed to look at thousands of genes). This is generally true regardless of the techniques used. If you're testing multiple genes, you adjust your p-values. If you're testing a single gene and have always only cared about that one gene, you use the p-value.
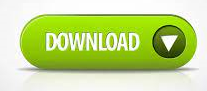